What I Learned at EDUCAUSE about the Higher Ed AI Conversation(s) Part 1
We are definitely not all having the same conversation...
Estimated reading time: 8.5 minutes
Don’t worry—if you don’t know what EDUCAUSE is, I’ll explain that too and I promise, this isn’t one of those posts that talk exclusively about a space you aren’t present in. Rather, it’s a natural space to talk about some of the larger trends and conversations happening.
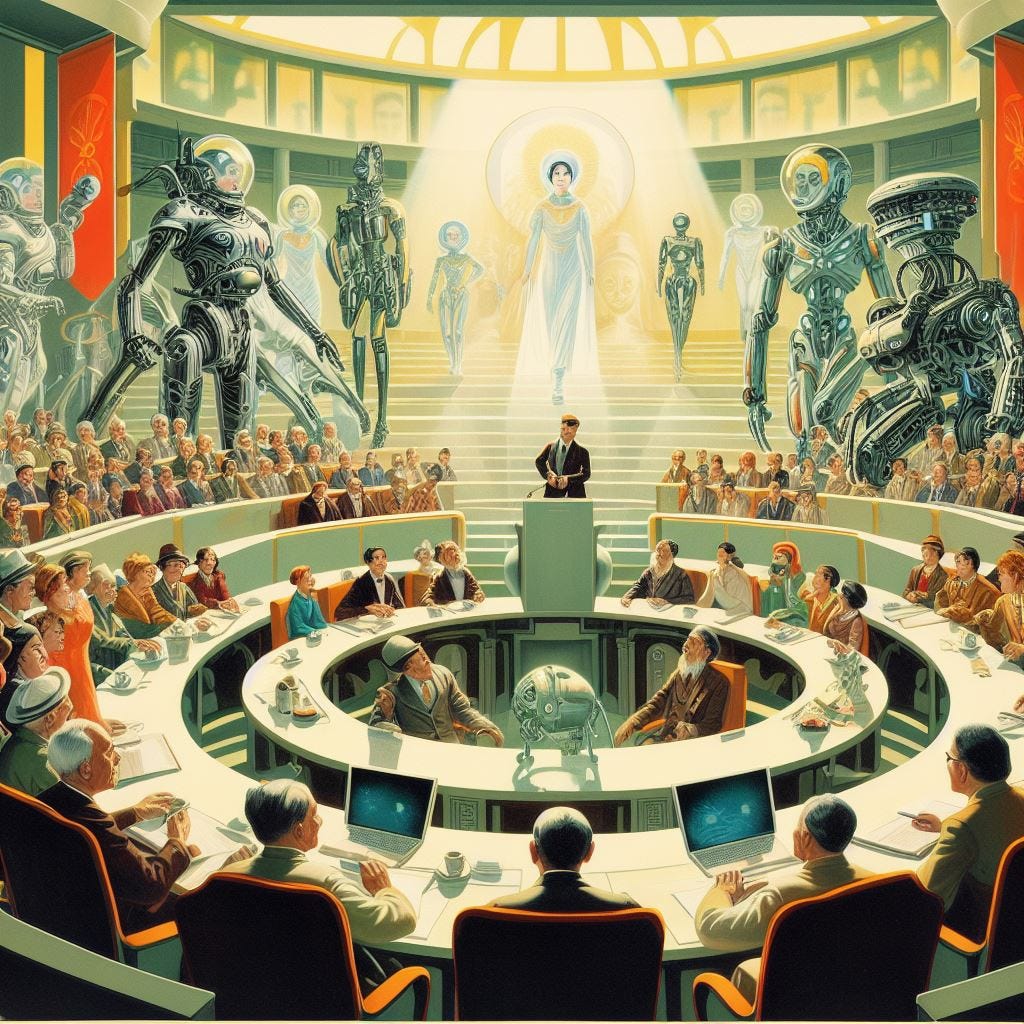
So I just attended my first EDUCAUSE conference. It’s a national organization that hosts an annual conference around education and technology. EDUCAUSE’s biggest claim to fame is that they issue the .Edu addresses to higher ed institutions.
I've been a frequent flyer of the NERCOMP conference which is the regional entity of EDUCAUSE in the northeast of the US. But the national conference is a whole other beast. They reported over 7,000 folks attending and it certainly felt like that. There was probably a ratio of vendors to institutional representatives of 1:1 or 1.5:1—there were a lot of vendors! But that’s the conference—a keynote or two, lots of presentations and poster presentations, and an exhibit hall with hundreds of vendors each with lots of folks wanting to talk to you about their AMAZING product.
This year, I attended because one of my students and I were invited to be on a leadership panel about generative AI in higher education. I was also doing a Poster Session on what we did at College Unbound (you can find my “visuals” here—I went with low-tech/visuals cause I just didn’t have the time or mind to do more). They offered my student a full scholarship and free conference admission for me, which then meant work could cover the rest.
Obviously, generative AI was on a lot of folks’ minds and many sessions were about it or it naturally came up as a result of the conversation. What follows isn’t a perfect summation but in the goal and pursuit of this project, I’m going to give readers a semblance of the different conversations that seemed to be going on across these spaces and from that, my own reflective analysis of what I saw. Of course, this is not comprehensive or perfect but I think it’s as good a place to start as any!
The Who, The What, & The What For
Everyone wanted to know about everyone else: who was using AI, what were they using, and what for. It felt a bit like a frenzied “show me yours and I’ll show you mine.”—Often to find that most of us had very little to show. That, in itself became a critique that folks bandied about as well. “Here we are, 11 months since the launch of the tool and its explosion everywhere and higher education with its snail’s pace approach to everything still has little sense of what’s going on.” (I don’t think that’s true, to be clear, but the commentary was present.)
That is probably the biggest challenge within all of this. It is hard to know who is doing what with what. Yes, lots of institutions are spinning things up or figuring things out, but few have launched much. The best example of an institution that seems to be doing something institutionally wide that is worthy of note is the University of Michigan with its internal AI tool.
But there’s no comprehensive or even reasonable collection of “here’s what’s going on at different places.” That would make for a great resource or newsletter. ITHAKA S+R is starting to look at the policy stuff and launch a project and EDUCAUSE is collecting higher education policies as well. But policies aren’t the same as learning what each institution is doing.
Without some central space to share these kinds of updates, I think there’s going to be a lot of reinventing of wheels—which is the thing that contributes to institutions being so slow. There’s so many other things higher education institutions are trying to do—trying to jumpstart, organize, and lead the challenges and possibilities of AI is just one more problem to figure out and determine.
Staffer, Researcher, Teacher, Student
Conversations were also focused on the who of it all. Obviously, the conversations about the classroom were abundant as they have been since last December but increasingly, the questions about researchers were raised (and they have been raised over the last 10 months too, but it felt more palpable), and finally, an increase of considering what about staff and how they can or should use it.
These challenges are part of what will and have been making it hard for institutions to get a grip on it. An institution has many components and not all of them operate under the same assumptions, motivations, values, or practices. How a university board may look to use or want AI used in the institution is going to be different from marketing to funding and development to admissions to student support. The different types of staff through a university also introduce lots of interesting use cases and lots of ways generative AI might be used.
Policy or Usage Guidance
During the panel I was on, a woman asked if the conversation should be about institutional policies or usage guidance. She elaborated that many parts of generative AI is already covered under other policies existing in the institution. Basically, she was asking do we really need more policy?
That’s a fair question, institutions are bloated with lots of policies. Just like in society, we have more laws than we know what to do with but are still responsible to “know” to avoid, in higher education, there are ample internal and external policies we must adhere to and integrate into our practice. So does AI warrant its own policy or does it just require adjusting or pointing to what already exists?
This is part of the challenge. Institutions need to do all three as far as I see it: Develop generative AI policy, adjust existing policy, and develop usage guidance. Let’s take these in reverse order.
The usage guidance can only come into place when there are policies in place (and tech leaders in higher ed can find ways of nudging and supporting that guidance with this recent article in EDUCAUSE that I co-wrote).
Adjusting existing policy also makes sense. For instance, this is what many colleges have done with their academic integrity policies. The language of such policies often did not include the language around generative AI. For instance, they would have language around acquiring a written paper through a paper mill or taking the words of another person. Generative AI is neither of these things so a student could make a technical argument.
There are definitely places where this makes sense to adjust to clarify the policy includes the specific elements of generative AI. There are also places where it’s self-evident that it exists and institutions just need to point to it clearly and loudly. FERPA, the law protecting the privacy of students is a good example where we can say unequivocally that generative AI falls under these laws and faculty should not enter private information of students into any generative AI.
But policy is still needed. In reality, that policy might not be grand and elaborate whereas the usage guidance might have more details and guidance. But policy feels right at this juncture because generative AI introduces the near-automatic creation of information and content—that is something that historically has been the role of individuals and is something particularly prided by folks within higher education.
Higher education is THE knowledge worker—creators and disseminators of knowledge. A new tool has arisen that appears to have similar and relevant abilities to produce information or content (some will argue knowledge—not sure I’m there yet) that feels strikingly similar to knowledge. It has implications for the knowledge we produce, the work that we do within and beyond the institution, and how we communicate.
Folks are finding ways of using it that reduce their workload and given how overburdened many folks throughout higher ed are—we can’t blame them—higher ed staff nearly always have jobs that are about 2-3 different jobs folded into one. Folks will need clarity about what is out of bounds for their institutional and individual protection. And because of the kinds of things that generative AI can do that previous tools can’t, folks are going to look to try lots of different things with it.
Not that they shouldn’t try but again, a thing brought up in many conversations is that we need to tread carefully to not reproduce harm or problems that we know have existed with previous technology. We are much more aware of the limitations, the immediate and long-term problems, and the legitimate concerns about generative AI from the get-go. Or, if not outright aware, folks should know that the conversation around generative AI has been robust—not just in the last 11 months but in the last 10 years so there is wisdom to glean and build upon as we prepare and craft policy to align with our legal obligations and institutional missions.
Ok—that’s part 1 from observations at EDUCAUSE. Coming up in future parts of this reflection, you can anticipate the following topics:
What We Know & How We Go
Institutional AI vs AI Being Used in the Institution
System Tools vs Copilot Tools
The Toybox
Strategies for Implementation & Education
Access, Costs, and Equity
AI+Edu=Simplified by Lance Eaton is licensed under Attribution-ShareAlike 4.0 International