What I Learned at EDUCAUSE about the Higher Ed AI Conversation(s) Part 2
More of the things I've learned & been thinking about
Estimated reading time: 8.5 minutes
In the last post, I dug into what folks are trying to figure out about generative AI, the different roles that higher ed has to think about regarding AI, and navigating the development of policies or usage guidance. This post will be a little more broader around the specific issues that are coming up.
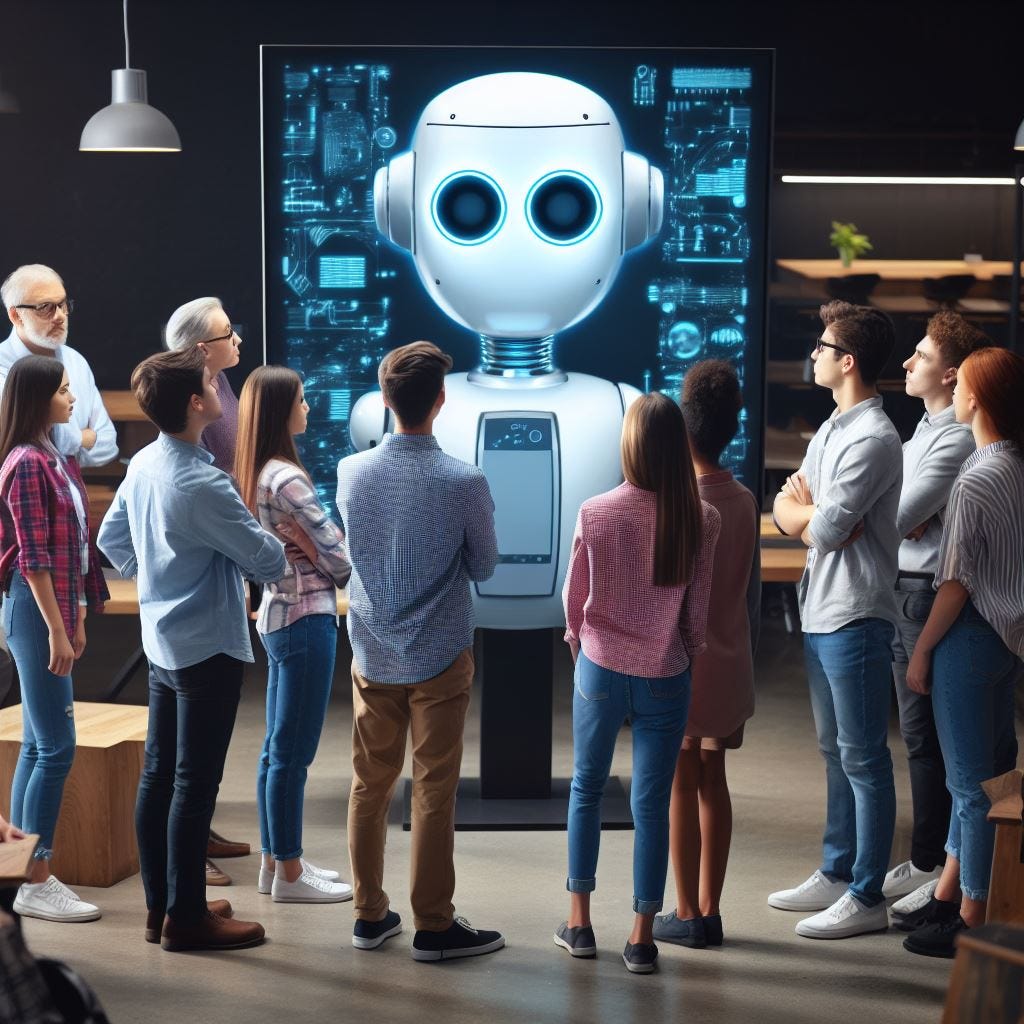
What We Know & How We Go
There’s a general confusion and angst about what we even know about generative AI collectively, and with that (or without that), how do we go forward? Part of what I saw at Educause is that nearly every vendor needed us to know about the AI elements of their tools. As Kate Miffitt, Director for Innovation at Cal State Univeristy, with whom I was on the leadership panel said, it feels like every company is touting its AI much like companies tout their gluten-free properties as if that was their original intention (I’m looking at you, “gluten-free” eggs!).
Part of a talk I’m giving next week—and I can’t believe I’m invoking Donald Rumsfeld—is to go through the known knowns, the known unknowns, and the unknown unknowns. It’s is a valuable way to talk about generative AI because there are definitely known concerns and known unknowns that we can anticipate, but we’re still wringing our hands about the unknown-unknowns. (And yes, I’ll be sharing that talk here, so stay tuned or subscribe if you haven’t yet!). And that is a significant part of the conversation.
While we’re not quite there yet, there was also a growing sense of “I’ll show you mine, if you show me yours” that seems to be permeating the conversation at the institutional level. That hesitation at showing, for most institutions, feels like institutions are trying not to so openly dawn the Emperor’s New Clothing. That is, there are a few institutions I think are doing robust and interesting things (or at least starting to) but most are saying they’re doing or starting to do things but nothing of substance. And that’s fine—there’s still a lot to sort out but folks are presenting themselves as really busy working at it when there might not be much to be working at yet.
Institutional AI vs AI Being Used in the Institution
A rich conversation going on is this difference between a college having an institutional AI tool. The best example of this is the University of Michigan system with its U-M GenAI tool that it has made available, which is widely available to different institutional members depending on the type of usage they are looking for. That is likely to be the case for some of the bigger institutions that they develop internal tools (or contract with existing generative AI entities).
A lot of institutions are going to be trying to figure out whether they are using institutional tools—tools that they have reasonable control over and can determine usage amounts or identify a range of acceptable tools and let individual departments decide which tool they want and how much they want to spend on it.
The first strategy is similar to institutional IT departments creating, maintaining, and determining bandwidth amounts for internet networks, even if they are working with outside vendors, they are the intermediary and standard bearer for all things internet networks. The second approach is creating the acceptable vendor list that departments can contract with and determining how much of their own budget they can or want to allocate.
While I think it will be smarter and more coherent to do the first strategy, that’s a heavy lift requiring more of IT departments. The second one is more likely and also likely to be a bit of a challenge for departments that are already struggling with lower budgets.
System Tools vs Copilot Tools
Along those lines, there’s an emergence of different generative AI tools that feel categorically different. Folks are looking for system-generative AI tools—tools that can work within the walled networks of the institution and move across the many different systems of an institution—from its student information system to is learning management system to its email system and the like, to help create insights, spot concerns, and bridge so much of the overflow of communications and reporting labor that must be done in an institution.
We’re not there yet but I can imagine that such system generative AI tools are what the bigger institutions are reaching for as they build their own generative AI systems or contract with the big players. The power of this approach would be the interoperability of a generative AI tool to work across the jangled mess of technology systems in a given institution—most of which barely play nice with one another and have a user-friendly component that is on par with the old model of the department of motor vehicles. System generative AI is the dream (and nightmare) that many folks think can change how the work gets done.
Yet, most generative AI for now is going to be co-pilots. Generative AI tools that show up in Google and Microsoft products. They’re not going to replace work but reduce the amount of time on things. They’re going to be part of the landscape of lots of different tools, but they are also not going to play well with others and stay within their own tool setting because of interoperability or rather the inability to cross-system interactions.
This is probably where we will start to see a new digital divide occur among highly-resourced institutions that will be primed or able to take quick advantage of general artificial intelligence (should it ever actually arrive on the scene) and the rest of higher education. These folks will then need to figure out how best to incorporate it (if they can afford or build the infrastructure quickly enough to do so).
The ToyBox
As I’ve mentioned here and in the first post, there are lots and lots of vendors ready to make money of the AI tsunami we seem to be experiencing. They want you to know they’re using AI, even if it isn’t generative AI but are largely using “traditional” AI (largely defined as AI created before generative AI such as voice-automated tools, automatic closed captions, and the like).
The challenge for all of us is that there are so many different folks selling so many different tools that it can be incredibly hard to sift through the bullshit that is being offered up. And it is unfortunately going to be like that for at least another year or two.
I read about 10-15 newsletters weekly on the latest in generative AI and many of them feature new tools that have arrived on the scene. And there are never not any new tools they have to share over the last 10 months.
Now, folks can explore them and learn more about all of them—and there are resources like those below to do so.
But if you’re like many of us, you’re too busy with too many things to figure it out. So what to do with this? Unfortunately, it is likely to go with the most popular ones (I’m increasingly finding Claude more interesting over ChatGPT) and learn about how to use that well. Inevitably, what you learn there will be transferable to others when the “great consolidation” happens.
What is the “great consolidation”? That’s when most of these tools get bought out by bigger companies or run out of venture capital with no real customer based or significant contribution to the generative AI tool cold war that’s going on right now.
Strategies for Implementation & Education
The EDUCAUSE conversation also raised lots of questions about what it means to implement generative AI in a given institution. The implementation has two conversations. If we choose tools, how will we deploy them effectively? The second and interrelated (but also separate for some places) question is how do we continue to educate about the usage of these tools?
Both conversations will need to involve IT, HR, the Chief Academic Officer, and several other key players at an institution, but should also include a more cross-functional team of folks to make sure sufficient questions and concerns are brought to the forefront.
The first question is going to hinge a lot on what can be afforded and maintained along with who will actually get to use it. The second question will need to be an ongoing project to support the usage of the tool and to keep an eye on the changes and developments of generative AI to figure out how to continue to update and navigate the support of all institutional members (faculty, staff, students, community members, 3rd party vendors, etc) that may be using or subject to use by these tools.
Up Next
Ok, I had opened to get to access, costs, and equity, but that’s turning out to be longer so I guess we’ll have a part 3. Stay tuned!
AI+Edu=Simplified by Lance Eaton is licensed under Attribution-ShareAlike 4.0 International