What I Learned at EDUCAUSE about the Higher Ed AI Conversation(s) Part 3
The final (?) entry where we talk about access, costs, and equity with generative AI & higher education
Estimated Reading Time: 8 minutes
All right, here is the final part (?) in this series around observations and reflections on conversations I had at EDUCAUSE this year. Check out part 1 and part 2 to get caught up.
There’s lots of different conversations that will happen around access, costs, and equity. That was quick clear from the various conversations. Let’s break those down a little bit before diving deep.
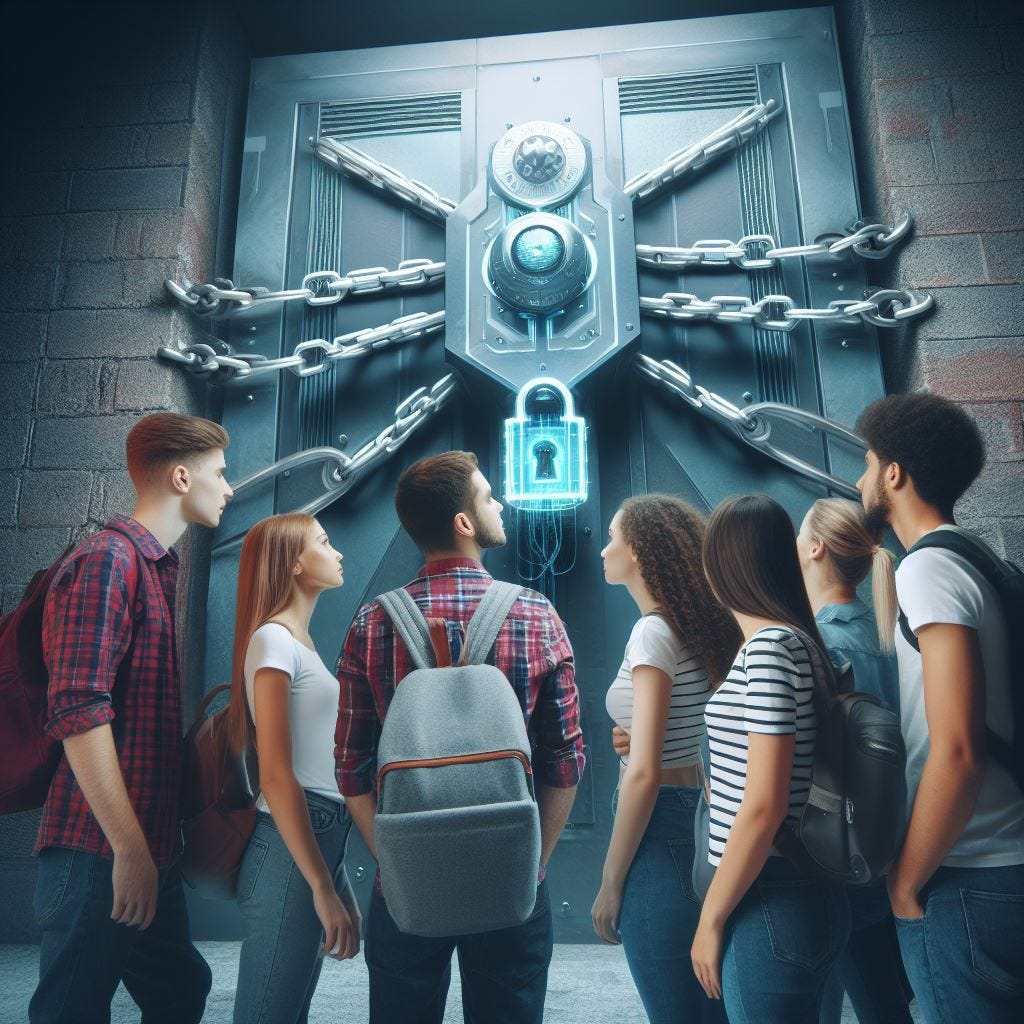
Access
When talking about access to generative AI in higher education, we’re talking about the different ways access can and may happen. Right now, there’s lots of “free” generative AI tools out there for anyone with internet access and how long will they remain free. These tools are costly to maintain and unlike social media, the revenue-generation opportunities by ads and such are not quite in place.
But access is and will continue to differentiate. Some generative AI tools have paid subscriptions like ChatGPT and Claude. These subscriptions open up more powerful models, additional features, and ways of using the tool.
With regards to higher education, there will be free-for-everyone tools (Bing, Google Bard, ChatGPT with GPT 3.5) and then there will be tools that institutions begin to offer to their institutional members just like they offer email, library access, and other software access.
Here, as I mentioned in part 2 is where we will see a new level of differing access in higher education. Some institutions will have institutional-wide AI systems and some will have copilots. Inevitably, Ivy League institutions will get their own institutional AI tools while places like community colleges will end up with copilots.
But even those institutional systems and copilots will have different layers just like subscription packages with cable where bundling will create different entertainment opportunities for each household. From this, access to generative AI will look different according to different institutions but, of course, it may get more complicated when it comes to costs.
Costs
A thing that took me a while to learn about higher education—like a ridiculous while to learn to the point that I could have some shame about it if I allowed myself to—is the idea that a college or university has different “schools” within it. I mean, I had heard these things like “The School of Arts and Science” or “The School of Medicine” but it flew right over my head as a part of the institutional structure to create small “schools” within a given college or university. I’m 92% sure that someone reading this is now learning that fact too.
But what I don’t think everyone in higher education also realize is that at different institutions, these schools are either centralized or decentralized (and yes, many are some hybrid of both). What that means is that a decentralized system largely recreates all the other parts of the institution (they may have their own IT system, their own center for teaching and learning, admissions team, etc). A centralized institution provides services across the institution into which each school draws. This eliminates duplicated services on the institution and also can cause issues in the specialization that some schools, given their size, may need.
These models also have different financial models to them in terms of how their budgets are created and allocated or used. For instance, they may get a budget but some portion of it may be to pay IT for its services in a centralized model. This is where costs come into play. Currently, generative AI is costly to operate at an institutional scale. Who is going to pay for what? Who gets how much usage?
That was some of what I started to hear being discussed at EDUCUASE. Institutions trying to figure out the right usage model. A simple and clear model is allowing everyone the same usage amount (equal usage). Others discussed a proportional approach to usage that either had to do with how much the given entity was a contributor or a drain on institutional resources (or overall mission). Others considered what would happen if each school or department paid for the amount they wanted (or could afford).
How institutions decide how to split and distribute the PAI (sorry—I had to!) will be a really interesting conversation over the ensuing years and I’ll circle back to parallels for us to think about this after the next section.
Equity
This process of figuring out the finance of generative AI will like create a new kind of digital divide. It’s not going to be people who have access and don’t have access but what kind of access do you have? I heard this question a lot at the conference around what generative AI means for institutional equity and the (failing) assumption of institutions as pillars of meritocracy.
Between the institutional systems vs the co-pilot access with the distributed cost and level of access to these tools creates different levels of access and availability that tell us it’s going to shake out poorly for lower-resourced institutions.
Institutional system tools will inevitably be more expensive and yet there will be a larger range of control and access that won’t be available to the co-pilot model. In the co-pilot model, institutions will also have to decide if they sort through the packages or do they allow for their individual areas, departments, and schools to do so. To do the latter will mean that even within institutions, there will be varying levels of access for students, faculty, and staff depending upon where you are in an institution.
This will extend an increasing inequity of higher education that will allow the benefits to further accrue in elite institutions while other institutions will struggle to keep up. There’s also possibilities to increase inequity across larger institutions about how can leverage these tools and who cannot.
One Thread for Consideration
Now, there are lots of inequities across higher education to which generative AI will affect, but for me, one place where it most strongly resonates is the knowledge production and dissemination mission of higher education.
Higher education’s two central goals are to create knowledge (research) and to disseminate it (teach). There should be more goals (and institutions do aim to expand beyond these) but these are the ones embodied in higher education for the last two centuries.
From the work that I’m doing for my dissertation (academic piracy in the 21st century), it’s increasingly evident that the knowledge production aspect of higher education is significantly impacted by the knowledge access problem. That is, scholars and students are limited in their abilities to access research literature in order to produce new research.
Somehow (ok, I know how, but it’s 60+ pages of my dissertation), higher education has created this system where it pays scholars to produce research, those scholars give that research away to publishers, and publishers sell it at unaffordable rates back to higher ed.
At many institutions, accessing research literature is a hard, tedious, laborious, and often fruitless process. It’s why there are academic pirate networks like Sci-Hub and LibGen where scholars go to download research literature (my dissertation will have more to say about why and their experience, but that’s a later conversation). And there are lots of scholars in the US and worldwide that are doing this. I’d send you to this article in Nature because it’s more contemporary—but it also costs $30 to access. So I’ll send you to this 7-year old article in Science because you can actually read it (and that, my dear readers summarizes the knowledge access problem—how many of you can freely access the first through your institutional affiliation or because you have $30 to blow on a single article).
What does this have to do with generative AI you ask? Generative AI is going to play a role in the research and production of knowledge. In fact, it will play many roles—some of which we’re stumbling into and others will become increasingly evident as we go along.
Two areas it’s going to influence research are in reducing the amount of time it takes to complete research and the ability to synthesize already existing research. (Note: this will look different according to each discipline).
Already, highly-resourced institutions already have a range of ways to make research happen more quickly. More access to research literature through their libraries, grad students, and a disproportionate amount of grant rewards to which their institutions are awarded to fund post-docs and the like. And generative AI will extend their production cycle, allowing them to more quickly create knowledge and get it out there.
Yes, generative AI will also help scholars at lower-resourced institutions, but again, scale and model favor the rich and continue to make it harder for scholars at lower-resources institutions both as graduate students and faculty to make the move into other institutions.
So that wraps up the conversation from EDUCAUSE, I’ll be going more into specific elements and concepts in the ensuing weeks. I also have a few talks lined up that I’ll be sharing the text and resources from for folks to benefit from. Thanks for reading!
AI+Edu=Simplified by Lance Eaton is licensed under Attribution-ShareAlike 4.0 International
I'm learning a lot here! Thank you. Don't totally understand yet why generative AI would exacerbate inequality between research institutions.
Your writing brought back memories for me of this Open Access Day in 2007 when I was working for the MIT Libraries. Students put stickers on journal shelves in the library showing the crazy high prices of journal subs. https://www.flickr.com/gp/nic221/G0v01N75e3